Traditional panels are dead! – Webinar recap
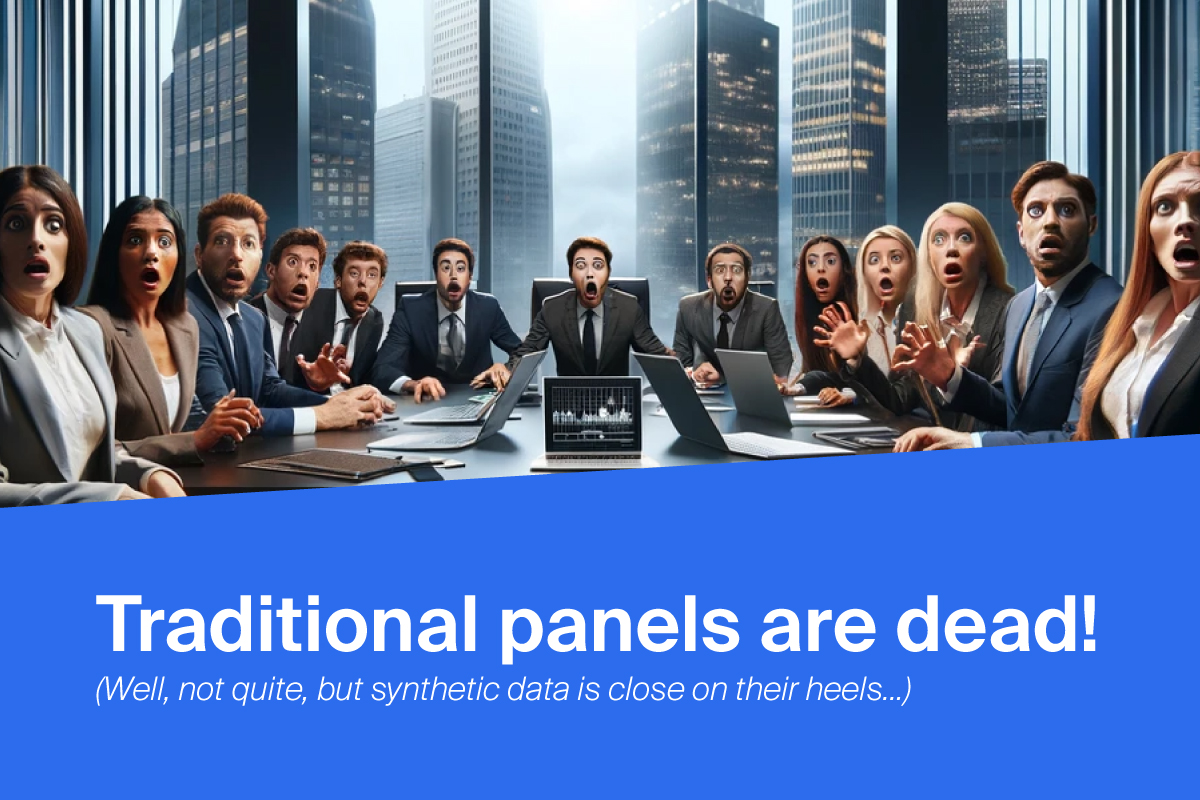
Contents
Of course, traditional panels aren’t truly dead – there is still a valid place for them as a part of your insights strategy.
But we’re in a new age of data creation. Let’s stop pretending that human opinion is the gold standard.
Below is a partial transcript of our recent webinar 'Traditional panels are dead!', presented by Doug Guion, an industry expert with over 25 years of experience in the data collection and market research space, who delves into:
- How synthetic data is stacking up against traditional sample across accuracy, relevancy, and quality of insight
- When and why panel research still holds its ground and how it can be effectively combined with AI-driven methods for unparalleled results
- How brands are using synthetic data TODAY to supercharge their insights
Explore the transformative role of synthetic data in market research with insights from Doug Guion’s webinar. Keep reading to uncover the potential, applications, and common misconceptions of synthetic data...
Traditional panels are dead!
All of the images from today's slides were generated through a chat GPT. There's lots of other ways to do it, but that's part of the fun of creating webinars now, being able to make your own images fit for purpose.
And why don't we go ahead and get started? Hi, everybody, my name's Doug Guion. Thanks a lot for coming today. We're here to talk about synthetic data in the market research space. And more specifically, an education in what's happening with synthetic data and where I see things going.
And I've been around in around the market research space for better part of 25 years. I’ve seen a lot of technologies come and go and I've never seen one generate as much negative reaction or fear-based reaction as I have with synthetic data.
So a lot of today is about hopefully opening people's minds. It's not to sell you on anything, it's not to try to convince you to change everything that you're doing. But skepticism, I expected, and even deep skepticism, I expected. But what I've been met with is contempt prior to investigation.
People saying, nope, it'll never work. This can't happen. And I think that's a, well, decidedly uncurious response to something that's really interesting from people who are naturally curious.
And I think it's perilous.
Today I want to talk a little bit about synthetic data in general because I'm finding that there's a lack of understanding or misunderstanding which leads to reservations about trying it. A bit about why this space is going to move or at least I believe it will move much more quickly than anything you've seen previously. Talking about the way that panels or online market research or information gathering is happening today. Some examples of how real brands are using virtual audiences or synthetic data to be able to answer business questions and then an overview of how it happens.
And more than anything, as we're all here, standing at the press base of technology that literally moves a thousand times faster than we do, there's three things I want to leave you with:
- Do I have an open enough mind to the prospect of what's coming and what's happening?
- Am I aware of how quickly it's happening and going to happen?
- And am I curious enough in my business in the way that I'm approaching things?
Those are the three things I think are the most important to engender regardless of what you ultimately do.
The shape of synthetic data
So first a little bit about synthetic data in general. There's a lot of different ways to talk about synthetic data. I'm going to talk about it in two different ways
First, AI-generated synthetic data without real-world data, without actual data – think of video games, etc. There are no artists that come up with these conceptions and you have to figure out what the physics of the players are going to be, what the landscape is going to feel like and look like and you can sort of do whatever you want. Synthetic data is used to create models that are then trained to be able to produce those. So that's a use for synthetic data.
And then you have something like synthetic data where you take actual data as an input, take advanced lung cancer screening as an example. You take patients who opt in to have their X-rays included into a data set, which then uses AI and machine learning algorithms to figure out the patterns from that data and what's presenting itself or not presenting itself.
Suddenly you come up with the ability to find early detection for cancer because you're able to project based on the synthetic data, what structures might develop if someone has cancer.
There's lots of real legitimate uses for synthetic data, but as you can see from the description, people often think of synthetic as being fake, like the computer is making something up. When really what's happening is you're using algorithms and processes to take a lot of the data that exists in the real world and act on it or transact on it in a way that makes it more useful and repurposes it.
It's not that it's false or it doesn't have a basis. It's just that the human mind isn’t able to do it nearly as quickly or as efficiently as AI is.
A short history of synthetic data
So, a very brief history. Synthetic data also as a class has been around since the 60s or the 70s.
I mean, it's, you know, 50, 60 years that it's been used in the early days. It was for nuclear testing, aerospace, defense, where it wasn't possible to test these things in the real world. Machine learning was employed to be able to do computer simulations, and it's continued to develop – obviously, nuclear is still a case for it.
But drug companies are using synthetic data to circumvent the need for the time that it takes for clinical trials or the expense of clinical trials. Autonomous vehicles are using synthetic data. So there's a host of ways that synthetic data has and is being used right now.
It's not brand new. This is something that's well established and has been accepted in a number of disciplines where the consequences are serious.
So synthetic data, again, as a class, is something that's been around for a long time. But where it's going is sort of a really enhanced degree of realism and complexity.
Think about something simple like virtually trying something on in a store where you can literally have an image of yourself and use AI techniques to see how a garment would feel and would look and would move with the physics of the room.
Integration with emerging technologies, autonomous vehicles is another one as well as IoT. So the Internet of Things, where every application in your house, every appliance in your house, all of the things around us are all going to start to be powered and driven and enhanced by AI in a nearly invisible way, but what it's going to do is make things easier and more enjoyable and provide more value more quickly.
No one knows how quickly this base is going to grow.
On the left, you can see there's a billion-dollar prediction. And other people are predicting 6 billion. No one is predicting that synthetic data won't grow, it's just how much and how quickly. And on the right, this shows how quickly those platforms got to both a million and then a hundred million users respectively.
You can see at the first branch it's you know a few hundred days and then at the second one it's a few years to five six years chat GPT got there in two months. It went from zero to a hundred million users in two months – again that doesn't necessarily on its own say that synthetic data and AI is going to become a dominant force, it’s just as a single data point. But it does show that interest in the trajectory of this particular market is huge and vast, and it's playing itself out in the data.
A note on Moore’s law and the pace of AI development
Speaking of pace, Moore's Law is something that said transistor power would double every couple of years. If you're not familiar with Moore, he created the company Intel that made all the computing chips that we still use in many of our computers.
The advancements and technology that you've seen before were largely governed by hardware or infrastructure needs. You had to have a faster processor, you had to have more energy, you had to be able to conserve more energy – to be able to have a big machine become smaller – so you could make it do more. There was a natural gating to how quickly things could progress. But AI isn't like that.
And when you look at it, there's been 272 years since Ben Franklin discovered electricity. When you go from flying a kite to the supercomputer and all of the stuff that happened in the middle, all of these are infrastructure dependent. You have to have electric cables, you have to have power sources, you have to have cathode ray tubes that are powered in this with a certain technology to be able to get TVs and so on.
All of the pace of development, whether it could have been fast or slower, they were all governed by infrastructure or hardware requirements. AI doesn't need that.
AI is algorithmically based, so creating novel algorithms where improvements to the algorithms as well as access to the data can mean that AI or synthetic data advancements could be a thousandfold as opposed to doubling every couple of years.
You could literally go from a model T to a range rover in one model year. And the question that I ask people is, if that were to happen, do you think that the buying pattern for the model T would stay consistent when you could get a range rover for the next year or six months later for the same price or less? And I would say no.
That's part of the proposition of synthetic data, which is, once you can establish and validate that it's working, if it can move a thousand times more quickly, are people going to make the same decisions that they're making today when the advent of this new technology makes a new decision possible?
On the validity of traditional panels
Some things that we know are true:
- People never lie
- They have perfect recall – they remember everything that they've done
- They never tell you anything false.
As a result, there are no technologies required to prevent respondent fraud. There are no ghost completes or bots or speeders, cheaters or Peters. There are no panelists who are on every single panel and take every survey just for money. There's none of that. And consequently, market research is always right. It's never wrong. Surveys are never programmed the wrong way. Questions are never missed. Scales are never flipped. And data's never processed incorrectly...
So, that hasn't been my experience. It might have been yours, but it wasn't mine. And the reason I bring this up isn't to lampoon it. But it's to showcase that market research as a practice is not perfect. So when people talk about the introduction of synthetic data and say it could never work – we're not replacing a perfect system that operates in a vacuum and has no flaws. We're talking about a hugely flawed process that has a lot of scaffolding built around it to make sure that the output is believable.
With the way that we build our sample frames and the programming that we do and the way that we write questions, all of that is routinized so that the artifact of data that's produced is trustworthy. Without those things, it's likely that you wouldn't be able to trust it.
So this is the point: the way that we're doing research today is recursive, circuitous, and error-prone. And we've made it work. There are a lot of systems in place to make work and it does work. But ultimately, there's a different way of doing it.
Start with the business question and instead of doing all of the tap dancing and the steps because you have to deal with questionnaires and probabilistic sample and people – if you can skip right to the insight, and get to that artifact of data that you can believe in, are you going to do it the same way?
And my premise is that as people start to get gain more comfort with how synthetic data is being produced and have their own personal experience with it, there's going to be many people who are going to today say that they won't try it, who are going to be quickly looking to adopt it.
Real examples of Virtual Audiences in action
Here's a couple of examples that have happened in the last couple of months, but without giving away company names:
This is a major media platform that everyone has heard of that has billions of users, and they do a monthly project where they look globally at pain points.
You know, what are users not happy about, what do they want to see, what isn't working. And it's expensive and it takes a while and you have to analyze it. It's a rear-view mirror because by the time you get the results, they're six to eight weeks old.
We did a test with virtual audiences and asked the same thing, right? What are the pain points with this platform?
In 20 minutes, we were able to replicate to a high degree of fidelity, the segmentation that they're using for their current audiences, and getting very similar feedback to validate what they're getting in the current online survey that they're doing.
This has now become something that they can do more often. Instead of having it be point in time, it can be always on. It can be all the time to be able to get that feedback to the product team more quickly.
A huge global CPG company wants to be able to iterate and test concepts more quickly.
So they sent three totally new concepts that had never been tested anywhere before that just came out of R&D. We were able to do concept test in 15 minutes where not only were able to then ultimately select the winner, but were also able to have conversations with the personas that were created that were discriminatory enough where they were getting a different answer and different tone of voice or a different quality of voice from the various personas that were created. Enough that they felt that this is something that they wanted to be able to move forward with.
There are people who are doing it right now, there are businesses who are starting to understand that not every question can be solved this way, but there's a lot of them that can.
So, why do people still fear synthetic data in research?
And still, people just don't like it, right? You know:
“All right, fine, you're doing it, but I just don't like it. It makes me uncomfortable. What does it mean? How do I have confidence?”
If you've read a research report on bad data, that's come about from synthetic data, it's likely that they use the large language model exclusively. They put something into ChatGPT or Bard or Llama, and they got something that had a recency bias that was way overly positive.
We don't do that at Yabble. We augment that data – we use the large language model as a global context layer, but we add global trend data and statistical data, review data, social media data – a host of sources, that address the topics and the questions and the personas that are created, which gets around a lot of the biases that I mentioned when you just use a large language model. It also ensures that you get much more diversity both culturally and demographically among the personas, because you're not going to a single source. You're going to a wide variety of global sources dynamically.
How does synthetic data stack up – from a researcher’s perspective
We're finding a very, very high degree of parity between things that are happening with traditional methods versus synthetic methods. And there are some places where it's shelf ready today:
- Top of the funnel ideation
- Behavior
- Thoughts
- Trends
All of that is ready today.
Certain things like testing visual concepts, or scalar questions, custom segments, or being able to test videos, it's not ready for that just yet. It's not ready to replace your brand tracker. If you understand the places where you can use it and do the right tests, you can very quickly prove to yourself the value.
My point is – start. I don't care what you do., whether you ever talk to Yabble again or you do something else to educate yourself. Think about the value that you provide to your customers and the way that you do that and is there the chance that synthetic data might be able to do that more quickly or more efficiently.
And find some examples where you can test that and prove it to yourself.
Getting started with synthetic data and Virtual Audiences today
If you're interested in getting started, if you want to try something out, get in touch with Yabble to try Virtual Audiences today.
This is going to go more quickly than any of us have ever seen before or are properly prepared for. It's best to not kick the can down the road and wait. Get started today and figure out a way of at least exposing yourself to what's possible so that you can start to have that be part of your go-forward strategy.
To watch the full webinar and see the Q&A portion of the session, visit the Yabble AI Academy and register to view our past webinars on-demand.